Delivering on a promise - Data Science, AI, ML and Big Data in Biotech
I am traveling back from biotech-hub Basel (Switzerland) with many impressions and as many questions from BioTechX 2022. A large European congress where data-lovers, -minds and -leaders from startups, like ours (knowing01), providers to (big) pharma connect and network!
Through process automation (although few very large pharma companies still fail at this point) and an advent of novel high-throughput measurement technologies the amount of data in drug discovery and disease research is exploding!
We all know, we need tools, automation and innovation to have a chance to get a hold of the value that remains hidden in the generated data - privately owned but also public. The diversity – let’s call it heterogeneity – of the data in biology and medicine is outstanding. While several standards exist for some data types, community standards are very often missing. Data should be FAIR, but very often isn’t.
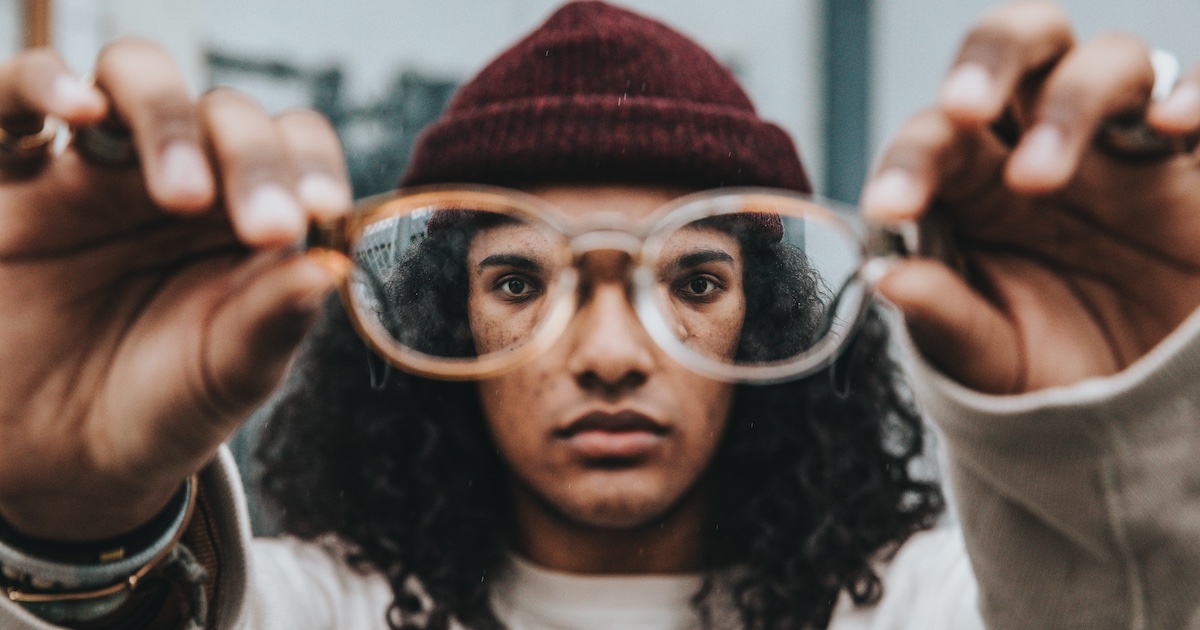
Is it enough to just analyse data?
While we all know, we need data scientists, bioinformaticians, machine learner, computer scientists, statistician and chemoinformatician and many more experts: It is not sufficient to – please apologise my language – “throw AI on data” to get an answer!
Because, YES, you guessed right: The answer to WHAT exactly? What was the initial question? Even better, we need to first understand: What is the problem we are trying to solve?
The problem assessment prior to the data generation and analysis, is very often not done. Hence its clear:
Much output was produced from heaps of data
– while the outcomes remain hidden.
Working as a data scientist, we know that for interdisciplinary teams meeting on eye level and sharing credits are the key to success and great outcome. Otherwise you are stuck with a hell lot of output.
Disappointments
Truly working in interdisciplinary teams often requires transformation of business processes and foremost a big shift of minds in many heads. Until the field entirely realizes this, the upper management might occasionally perceive that data science service units still need to proof their value.
Yes, data scientists need to become more product and outcome oriented. Yes, organisations need to embrace a culture of collaboration of equals. We - as field of data-driven research in biotech - won’t succeed without.
Big challenges
Digital transformation is necessary but not yet enough: A new culture of collaboration between business, product and data science units on eye level need to establish.
Data Standards are important for future data usage, but are often neglected as it feels like an overhead work.
Innovation – like ours – need to be pushed to the market in order to seamlessly equip organisations to concentrate on the outcome generation and collaborate with experts for specific data tasks.
This, and for sure a lot much more, will enable companies and big organisations to being able to bring the right data to the right people in the organisation.
Photo Credits: Balloon on street photo and woman holding glasses photo by Nathan Dumlao on Unsplash. Hands on tree photo by Shane Rounce on Unsplash.